MLOPS Solutions
Advanced MLOps Consulting. Streamlined Strategies for Model Deployment and Infrastructure Optimization
MLOps at Scale
Our MLOps solutions enhance governance in your AI operations. Expand, centralize, manage, and meet regulatory standards. implement security best practices tailored to your unique needs.
They trust us
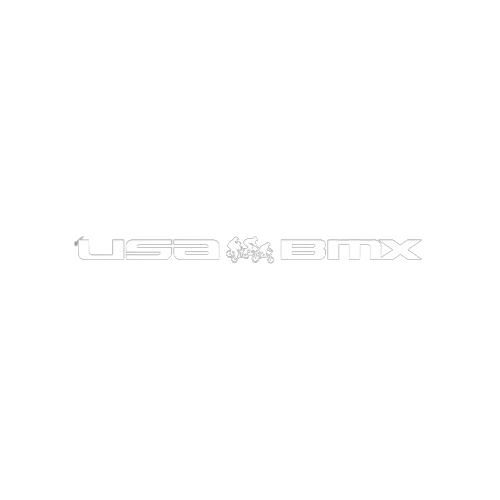
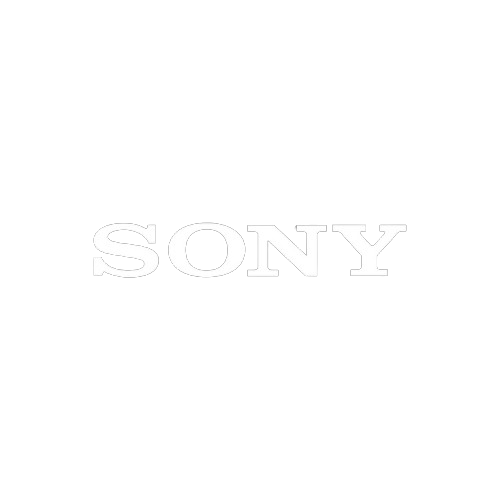
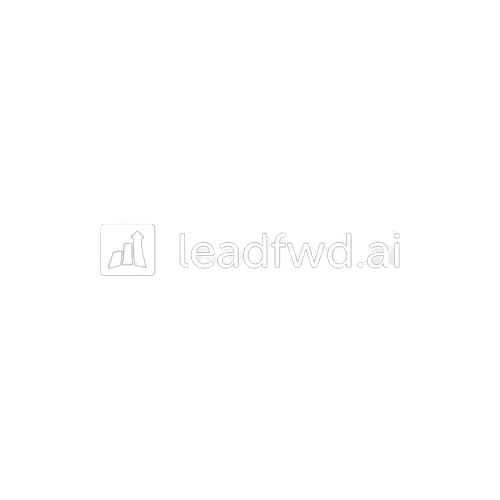
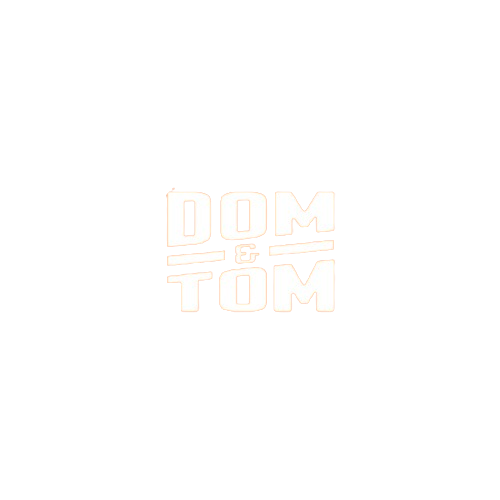
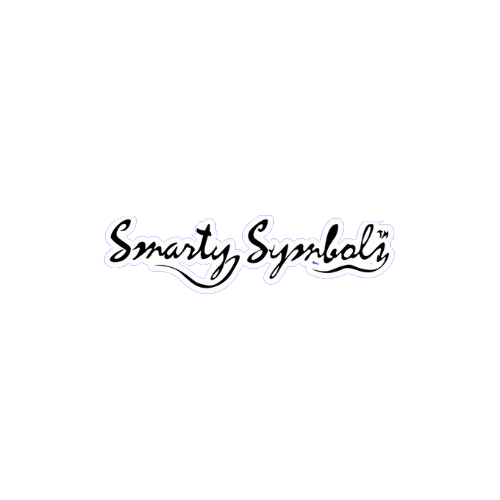
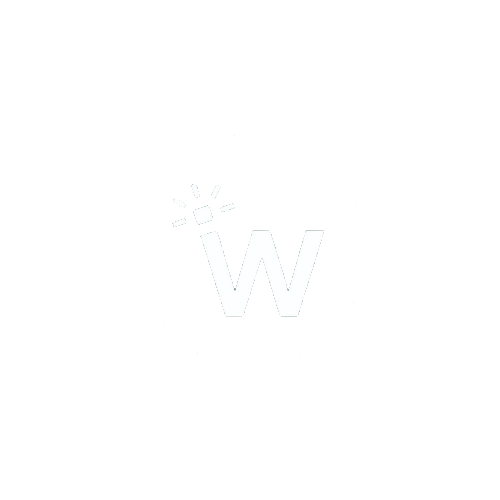
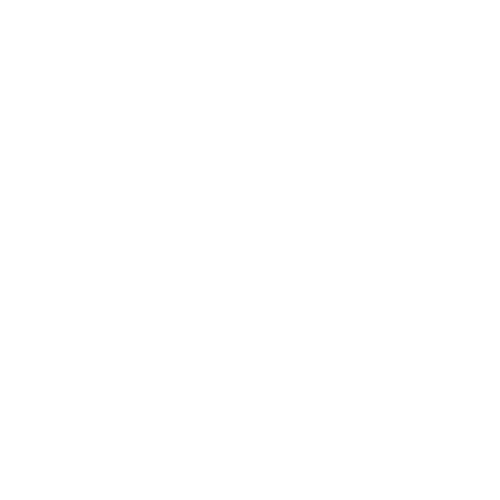
MlOps Capabilities
Management of Models
Simplify the management of your AI assets. Catalogue, monitor, and enhance reproducibility.
Scaling Artificial Intelligence
MLOps excels in scaling AI, from extensive experimentation to large-scale deployments.
Model Deployment
Automatically deploy to production, support various protocols, scale up to meet demand.
Unification
Create a uniform environment can enhance governance and increase efficiency.
Expandable Workflows
Adjust your data ingestion or training pipelines to suit your needs.
Monitoring and Alerting
Preserve your model's integrity with monitoring and alert systems.
FAQ
MLOps consulting focuses specifically on the operationalization of machine learning (ML) models, integrating data science workflows with DevOps practices. While DevOps primarily deals with software development and deployment, MLOps extends this to include the unique
MLOps consulting establishes best practices for model monitoring, automated retraining, and deployment pipelines, ensuring that AI models perform reliably and can scale efficiently as demand grows. By implementing continuous integration and delivery (CI/CD) pipelines tailored to ML workflows, organizations can accelerate the deployment of high-quality models.
The MLOps consulting process typically begins with assessing the current state of ML infrastructure and workflows within an organization. This is followed by designing and implementing robust pipelines for data preprocessing, model training, evaluation, and deployment. Continuous monitoring and optimization ensure that models remain effective in real-world scenarios.
MLOps consulting helps organizations establish governance frameworks that ensure compliance with regulatory requirements and internal policies. This includes tracking model lineage, documenting model performance metrics, and implementing access controls to protect sensitive data used in ML processes.
Common challenges in MLOps implementation include managing complex data pipelines, selecting appropriate tools and technologies, and bridging the gap between data science and operations teams. MLOps consulting services provide expertise in designing scalable and reliable infrastructure, selecting suitable ML frameworks, and fostering collaboration between cross-functional teams to overcome these challenges effectively.
Start Your mlops development proyect now
Get in touch today and receive a complementary Consultation